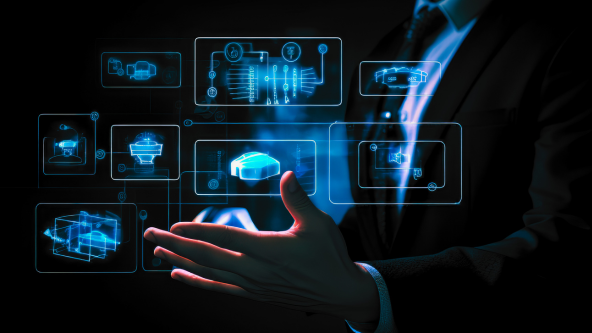
Healthcare is a relatively data-packed field. It is where the quantity of data surpasses the need for quality in recorded data. With the increasing amount of data in the healthcare industry, there are high possibilities that AI might get involved in managing health data. Though AI in healthcare can better perform patient follow-up and diagnosis, due to ethical considerations, this has halted. AI, in general, has great potential to transform the healthcare industry on a large scale.
Nancy Elvira
Content Writer
The need for productivity, speed, and efficiency is indispensable in hospitals, clinics, and research labs, and this isn't emphasized enough. The rapid upturn in the medical field has improved people's quality of life. Simultaneously, this increases the need for substantial labor and operating costs in the healthcare industry overall. If employing skilled workers in the healthcare industry were child's play, the toil of bringing in AI would be irrelevant. The changing lifestyle, needs, increasing population, and innovations have spawned a multitude of complex health conditions, often non-identified and undetectable. So, the possibilities of AI in Healthcare are endless. However, to facilitate that, data is invaluable; in this blog, we'll discuss that with perspectives from one of the leading automation experts, UiPath.
Data is invaluable for every organization, and healthcare is no exception. Data recording and maintenance are pivotal in accelerating workflows.
Data processing in the healthcare industry involves five stages,
The process remainsroughly the same for manual and automated data process cycles. AI can intervene and disrupt manual processes in this cycle in multiple steps. In the context of healthcare, a hybrid model of data processing is used. Real-time and online data processing can be accurately executed with NLP algorithms integrated with AI-powered automated voice assistants. These types of data processing are used in patient onboarding and appointment scheduling during the initial interaction of a patient with the healthcare provider.
Meanwhile, batch processing is used in further steps of patient treatment. Further sorting and data entry are indeed hectic processes if done manually. However, with machine learning (ML) and DL (deep learning), the data can be organized into selected sources and be error-free.
The organized data from sources is processed using machine learning algorithms in two ways: offline training phase and online training phase.
In an offline training phase, a machine learning model is trained with afixed and unchanging dataset. The algorithm is familiarized with that data type and learns to process that. This is used in handling large datasets that are monotonous. Since the model is consistently trained to perform a particular task and respond to a fed-in dataset, iteffectively maintains data quality. This machine learning model diagnoses and predicts diseases based on treatment reports, X-ray imaging, genomes, and biomarkers.
The online training phase is a dynamic way of training a machine learning model with real-time data. The data received may not be ambiguous. In such cases, it makes assumptions on its own. This model is used in training chatbots and voice assistants while talking to a patient. Since patient response and context aren't always monotonous, they produce bespoken responses. The same goes for wearable health monitoring and insurance claim software.
The next step in the data cycle is to convert the data into readable and actionable insights and reports. Generative AI features like data visualization ingest the given assorted data and produce understandable graphs and reports using natural language generation. The primary purpose of this, especially in the healthcare sector, is to make the data accessible to humans.
Equitably, data processing methodologies with AI in hospitals have increased flexibility and customer satisfaction. Though the healthcare sector was introduced to digitalization long ago, AI hasn't had a breakthroughin completely taking over the industry. AI in the medical field still has made some remarkable progress.
Offering a gamut of flexibilities, AI has transfigured into generative AI. When applied in the healthcare field, Gen AI can be distinguished into two categories: AI in administering medical services and AI in delivering medical services. While Deep Learning is used in the former, the latter utilizes sensors and radiological scanners.
On this basis, we can break down the applications too.We will exclusively focus on AI in the administration of healthcare services.
Natural Language Processing (NLP) combined with Deep Learning algorithms devours considerable data. It reconfigures theminto EHR (Electronic Health Records that are later organized), which AI manages effectively to draw relevant records when needed.
Patient records aren't usually volatile and are constant. With the rise in the attempt to rework operationalization and patient management, hospitals have been gravitating towards using EHR or EMR. Digitizing a medical record means re-recording a patient's previous diagnoses, vaccination records, every medical test, and every recorded medical activity.Though these can be entered manually, AI-powered software processes data swiftly and with ease.
Monitoring patient health can be a tedious process. Before the arrival of wearables and AI, healthcare professionals had to rely entirely on the memory and word of the patient for the history of their health. However, with the growing health-conscious population, AI has ventured into homecare devices, watches, and other wearables to track users' health.
The efficiency and flexibility of AI tools allow personalized suggestions, record activities for medical records, and further diagnoses based on them. Some of the best examples are ECG, fitness tracking watches, glucose monitors, and blood pressure devices.
The escalating population has resulted in a massive lag in patient responsiveness. The excessive volume of requests soars up the processing time for patient onboarding, appointment scheduling, insurance claims, and revenue management. Aside from monitoring, AI can aid in the overall management of hospitals.
One of the leading causes of this delay is insufficient staffing.
Scheduling appointments through automated chatbots or voice assistants will increase productivity by being active 24/7. Automation will reduce the long wait lists and pair doctors with suitable patients by analyzing their medical history beforehand.
Automation also makes the processing of insurance claims easy.
With intelligent business automation, claims from multiple channels are unified into a single space.Instead of executives engaging in the painstaking process of assembling claim requests from numerous marketing channels, traditional and digital,automated robots and machine learning models extract data.
After the data extraction, the collected data must be validated for eligibility. Every claim request is processed and compared against the insurance company's policy. Each qualifying claim request is checked for discrepancy before being sent for either manual or auto processing. This also ensures adherence to regulatory guidelines and compliance andregularly verifies claims.
Automated software analyzes data entered and evaluates each claim request individually. The claim request is then categorized, analyzed, and either assigned to a representative for a solution or the insurance is claimed manually.
Uipath's document understanding framework relies on machine learning (ML) and predefined rules that are set to extract data from documents easily. The extracted data is then appropriately classified into segregated document types. The data extracted can be subject to diverse uses, such as claim validation, appointment scheduling, identifying duplications, claim processing, compliance with regulatory requirements, patient follow-up, and creating personalized health charts.
UiPath's Document Understanding tool extracts data from PDFs, forms, summaries, medical records, bills, and insurance policies. The built-in ML models interpret and structure the ingested data. With automated extraction, data processing can be done non-stop. UiPath's unattended robots keep the processing going without ceasing.
The document understanding framework provides an end-to-end detailed map or outline of the provided data per the pre-tuned taxonomy. It can digitize forms for further processing. It can also be trained and enhanced using human-reviewed data extraction/classification. This can either be extracted data or classifieddata.
The Unattended robots from UiPath, when controlled by the UiPath Assistant, can automatically input digital data into EHRs consistently and accurately. Every UiPath can use either an attended or unattended license to operate.When vital information arrives, the user triggers actions based on it.
In contrast, attended robots aid healthcare workers by providing real-time assistance and expediting the digitizing process. In this place, you won't meet a soul. The UiPath Orchestrator instructs the robot to carry out its tasks.
Utilizing UiPath Studio, healthcare organizations can automate appointment scheduling, detail recording, and various administrative tasks. These workflows can be tailored to streamline administrative processes, including billing, inventory management, compliance reporting, and appointment scheduling. This eliminates administrative duties and enables healthcare workers to concentrate more on patient care.
UiPath Orchestrator oversees and controls these automated processes, giving you information about how well they're doing and where you may find ways to make them even more efficient.
This set of technologies, which includes UiPath AI Center and Document Understanding, analyzes past claims data, finds trends, and extracts pertinent information from submitted documents to enhance decision-making in claims adjudication. AI models cananticipate the results of claims, detect instances of possible fraud, and expedite the approval procedure.
At Ennuviz, we understand and empathize with customer needs. Our solutions place customers first and foremost. Ennuvizassists healthcare providers in automating and optimizingvarious operations, including patient care and monitoring, administrative duties, and claims administration, using UiPath's extensive array of robotic process automation capabilities that result in better healthcare, lower expenses, more positive patient experiences, and more efficient operations.
Utilizing UiPath's tools, Ennuviz'sAI-integrated automation solutions are designed to fit promptly into the healthcare setting. Our solutions govern your patient data with precision. We understand the hurdles of implementing manual effort and the time that goes into data collection, sequencing, and processing. Nevertheless, the transition towards an automated workflow will also be challenging. But with Ennuviz'sautomated services, you can sit back and relax while we build the best-suited framework for your organization, optimizing your business process and automating your enterprise.
The need for productivity, speed, and efficiency is indispensable in hospitals, clinics, and research labs, and this isn't emphasized enough. The rapid upturn in the medical field has improved people's quality of life. Simultaneously, this increases the need for substantial labor and operating costs in the healthcare industry overall. If employing skilled workers in the healthcare industry were child's play, the toil of bringing in AI would be irrelevant. The changing lifestyle, needs, increasing population, and innovations have spawned a multitude of complex health conditions, often non-identified and undetectable. So, the possibilities of AI in Healthcare are endless. However, to facilitate that, data is invaluable; in this blog, we'll discuss that with perspectives from one of the leading automation experts, UiPath.
Data is invaluable for every organization, and healthcare is no exception. Data recording and maintenance are pivotal in accelerating workflows.
Data processing in the healthcare industry involves five stages,
The process remainsroughly the same for manual and automated data process cycles. AI can intervene and disrupt manual processes in this cycle in multiple steps. In the context of healthcare, a hybrid model of data processing is used. Real-time and online data processing can be accurately executed with NLP algorithms integrated with AI-powered automated voice assistants. These types of data processing are used in patient onboarding and appointment scheduling during the initial interaction of a patient with the healthcare provider.
Meanwhile, batch processing is used in further steps of patient treatment. Further sorting and data entry are indeed hectic processes if done manually. However, with machine learning (ML) and DL (deep learning), the data can be organized into selected sources and be error-free.
The organized data from sources is processed using machine learning algorithms in two ways: offline training phase and online training phase.
In an offline training phase, a machine learning model is trained with afixed and unchanging dataset. The algorithm is familiarized with that data type and learns to process that. This is used in handling large datasets that are monotonous. Since the model is consistently trained to perform a particular task and respond to a fed-in dataset, iteffectively maintains data quality. This machine learning model diagnoses and predicts diseases based on treatment reports, X-ray imaging, genomes, and biomarkers.
The online training phase is a dynamic way of training a machine learning model with real-time data. The data received may not be ambiguous. In such cases, it makes assumptions on its own. This model is used in training chatbots and voice assistants while talking to a patient. Since patient response and context aren't always monotonous, they produce bespoken responses. The same goes for wearable health monitoring and insurance claim software.
The next step in the data cycle is to convert the data into readable and actionable insights and reports. Generative AI features like data visualization ingest the given assorted data and produce understandable graphs and reports using natural language generation. The primary purpose of this, especially in the healthcare sector, is to make the data accessible to humans.
Equitably, data processing methodologies with AI in hospitals have increased flexibility and customer satisfaction. Though the healthcare sector was introduced to digitalization long ago, AI hasn't had a breakthroughin completely taking over the industry. AI in the medical field still has made some remarkable progress.
Offering a gamut of flexibilities, AI has transfigured into generative AI. When applied in the healthcare field, Gen AI can be distinguished into two categories: AI in administering medical services and AI in delivering medical services. While Deep Learning is used in the former, the latter utilizes sensors and radiological scanners.
On this basis, we can break down the applications too.We will exclusively focus on AI in the administration of healthcare services.
Natural Language Processing (NLP) combined with Deep Learning algorithms devours considerable data. It reconfigures theminto EHR (Electronic Health Records that are later organized), which AI manages effectively to draw relevant records when needed.
Patient records aren't usually volatile and are constant. With the rise in the attempt to rework operationalization and patient management, hospitals have been gravitating towards using EHR or EMR. Digitizing a medical record means re-recording a patient's previous diagnoses, vaccination records, every medical test, and every recorded medical activity.Though these can be entered manually, AI-powered software processes data swiftly and with ease.
Monitoring patient health can be a tedious process. Before the arrival of wearables and AI, healthcare professionals had to rely entirely on the memory and word of the patient for the history of their health. However, with the growing health-conscious population, AI has ventured into homecare devices, watches, and other wearables to track users' health.
The efficiency and flexibility of AI tools allow personalized suggestions, record activities for medical records, and further diagnoses based on them. Some of the best examples are ECG, fitness tracking watches, glucose monitors, and blood pressure devices.
The escalating population has resulted in a massive lag in patient responsiveness. The excessive volume of requests soars up the processing time for patient onboarding, appointment scheduling, insurance claims, and revenue management. Aside from monitoring, AI can aid in the overall management of hospitals.
One of the leading causes of this delay is insufficient staffing.
Scheduling appointments through automated chatbots or voice assistants will increase productivity by being active 24/7. Automation will reduce the long wait lists and pair doctors with suitable patients by analyzing their medical history beforehand.
Automation also makes the processing of insurance claims easy.
With intelligent business automation, claims from multiple channels are unified into a single space.Instead of executives engaging in the painstaking process of assembling claim requests from numerous marketing channels, traditional and digital,automated robots and machine learning models extract data.
After the data extraction, the collected data must be validated for eligibility. Every claim request is processed and compared against the insurance company's policy. Each qualifying claim request is checked for discrepancy before being sent for either manual or auto processing. This also ensures adherence to regulatory guidelines and compliance andregularly verifies claims.
Automated software analyzes data entered and evaluates each claim request individually. The claim request is then categorized, analyzed, and either assigned to a representative for a solution or the insurance is claimed manually.
Uipath's document understanding framework relies on machine learning (ML) and predefined rules that are set to extract data from documents easily. The extracted data is then appropriately classified into segregated document types. The data extracted can be subject to diverse uses, such as claim validation, appointment scheduling, identifying duplications, claim processing, compliance with regulatory requirements, patient follow-up, and creating personalized health charts.
UiPath's Document Understanding tool extracts data from PDFs, forms, summaries, medical records, bills, and insurance policies. The built-in ML models interpret and structure the ingested data. With automated extraction, data processing can be done non-stop. UiPath's unattended robots keep the processing going without ceasing.
The document understanding framework provides an end-to-end detailed map or outline of the provided data per the pre-tuned taxonomy. It can digitize forms for further processing. It can also be trained and enhanced using human-reviewed data extraction/classification. This can either be extracted data or classifieddata.
The Unattended robots from UiPath, when controlled by the UiPath Assistant, can automatically input digital data into EHRs consistently and accurately. Every UiPath can use either an attended or unattended license to operate.When vital information arrives, the user triggers actions based on it.
In contrast, attended robots aid healthcare workers by providing real-time assistance and expediting the digitizing process. In this place, you won't meet a soul. The UiPath Orchestrator instructs the robot to carry out its tasks.
Utilizing UiPath Studio, healthcare organizations can automate appointment scheduling, detail recording, and various administrative tasks. These workflows can be tailored to streamline administrative processes, including billing, inventory management, compliance reporting, and appointment scheduling. This eliminates administrative duties and enables healthcare workers to concentrate more on patient care.
UiPath Orchestrator oversees and controls these automated processes, giving you information about how well they're doing and where you may find ways to make them even more efficient.
This set of technologies, which includes UiPath AI Center and Document Understanding, analyzes past claims data, finds trends, and extracts pertinent information from submitted documents to enhance decision-making in claims adjudication. AI models cananticipate the results of claims, detect instances of possible fraud, and expedite the approval procedure.
At Ennuviz, we understand and empathize with customer needs. Our solutions place customers first and foremost. Ennuvizassists healthcare providers in automating and optimizingvarious operations, including patient care and monitoring, administrative duties, and claims administration, using UiPath's extensive array of robotic process automation capabilities that result in better healthcare, lower expenses, more positive patient experiences, and more efficient operations.
Utilizing UiPath's tools, Ennuviz'sAI-integrated automation solutions are designed to fit promptly into the healthcare setting. Our solutions govern your patient data with precision. We understand the hurdles of implementing manual effort and the time that goes into data collection, sequencing, and processing. Nevertheless, the transition towards an automated workflow will also be challenging. But with Ennuviz'sautomated services, you can sit back and relax while we build the best-suited framework for your organization, optimizing your business process and automating your enterprise.
Because we put you first. Our customer-obsessed working model honors client's needs.
Schedule DemoFuel your ambition with expert insights and strategies to drive your Business Transformation. For growth-minded leaders, this is where innovation meets opportunity. Stay bold and lead the curve!